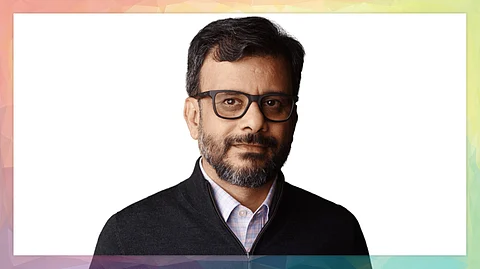
As AI reshapes the business landscape, the true kingmakers are not the models themselves, but the quality and exclusivity of the data powering them. A tech revolution like AI unleashes rapid change, and not every firm or job will survive.
While the large firms creating massive AI models, such as OpenAI and Anthropic, undoubtedly have a good shot at success, the rest of the businesses that innovate, compete, and profit from this revolution will be the ones with the best data and not the best models.
What defines “better” data? That is entirely subjective. Better data for your company is confidential, copyrighted, personal, or otherwise exclusive and tailored for your industry or use case. We call this “proprietary data,” and it is nothing new.
I’ve been an entrepreneur and CTO since the dotcom era when machine learning (ML) was synonymous with statistics, and proprietary data has always been at the heart of my business models.
One lesson I’ve learned from successfully building multiple data+AI companies is that simply owning a proprietary data asset is not enough. For example, a medical institution may use its proprietary patient data, medical records, and treatment outcomes to build Generative AI applications to assist clinicians. Publishing companies may create personalized learning applications using their proprietary content available in books, articles, and images.
Time and time again, I come back to famed computer scientist Peter Norvig’s axiom: “More data beats better algorithms, but better data beats more data.” It’s time business leaders take this to heart, too.
The most competitive firms in the age of AI will have “better data.” They will understand and segment data by quality before feeding it into a model and evaluate the new model's performance after the fact. Crucially, a collaborative culture will elevate a firm's capacity to fully exploit proprietary data in this evolving landscape of data-centric AI.
Stakeholders must stay aligned toward the business goal while ensuring the AI solution is robust, ethical, and unbiased.
The rapid adoption of generative AI has accelerated the transition of AI and ML product development from model-centric AI to data-centric AI. Business leaders have to keep up. Instead of blindly launching a search for the “right model,” leaders should put first things first and ask themselves, “Do we have the right data?”
Here is an easy way to understand the distinction between the two:
Instead of asking how to adjust the model to deal with the noise in a particular dataset, we should ask how we can understand, modify, and improve the data so trained models deliver the desired business outcome.
Why does this matter?
Foundation models, or Large Language Models (LLMs) are trained mainly on publicly available internet data and excel at expressing general topics. What these large models can do is impressive, but they fall short on tasks that require leveraging confidential, copyrighted, personal, or exclusive data.
It’s like expecting a high school graduate to ace a graduate-level calculus test without any preparation. Unfortunately, it is precisely this type of data that is so important for businesses and customers. But there are methods to take an off-the-shelf foundational model from the likes of OpenAI, Anthropic, or Meta and fine-tune it for specific needs.
When a foundational model needs to learn from a proprietary dataset, we use a technique known as "fine-tuning.” This process trains the model on a smaller, high-quality, and task-specific proprietary dataset to complement its broader general knowledge with specialized information to handle specific use cases. It is as if we gave personalized 1:1 tutoring to someone who has only ever attended massive lecture classes.
The difficult part of fine-tuning almost always lies with the data used to train the model. Fine-tuning is as much about the quality of the underlying data as it is about the model itself. By identifying high-quality diverse data points in the dataset used to train the model, models generalize better to unseen inputs and deliver accurate outcomes.
However, managing data for machine learning involves optimizing computational resources, understanding data distributions, and preventing overfitting while adapting to new data distributions.
When fine-tuning, companies must identify which parts of the proprietary data asset are representative and high-quality for the model. We call this “data evaluation;” while it requires effort up front, that work pays off by significantly reducing model training time and cost.
Think of data evaluation as an essential step so that better models can be trained to derive desired value from AI. The outcome of the data evaluation process is a new, high-quality version of the original dataset that is ready for action.
Implementing AI to transform your business extends beyond merely choosing an off-the-shelf tool. It's an interdisciplinary endeavor that spans algorithms, data engineering, domain expertise, ethics, and user experience.
Generative models are powerful, but the flip side of that power is a weakness. The wide range of possible responses makes it difficult to exhaustively evaluate model behavior across all potential inputs. Traditional model-centric metrics, such as accuracy or loss, do not capture the nuances of generative tasks.
The checks a firm needs are task-specific to ensure correctness, appropriateness, and truthfulness. The highly tailored, precise nature of proprietary knowledge means that, on top of data evaluation, firms must also ensure quality at the latter end of the process before a model reaches its end users. Aligning the business and technical stakeholders across this end-to-end process demands unprecedented cross-functional collaboration.
The bottom line: AI is a team sport with multiple players across product, data science, engineering, and the knowledge workers who are often the end users. Leaders must enable practitioners with systems of intelligence and organizational knowledge that allow them to improve data.
In this new AI landscape, those who harness the potential of proprietary data and foster a culture of collaboration will lead the way—those who don't risk obsolescence.
About the Author:
Vivek Vaidya is CEO of MarkovML, a collaborative, data-centric AI platform, and Founding General Partner of data+AI startup studio super{set}. Before super{set}, Vaidya was CTO of Salesforce Marketing Cloud. He holds an MS in Mathematics and Computer Applications from the Indian Institute of Technology, Delhi, and an MS in Computer Science from the University of Denver.