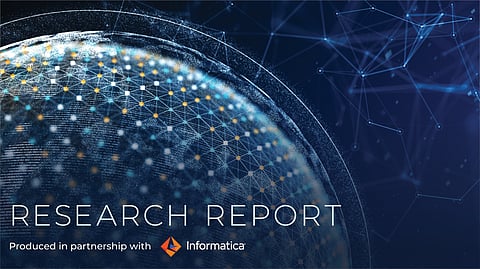
“How AI-ready is my organization?”
This question has most likely crossed the minds of all forward-thinking enterprise data leaders at least once over the last several months.
We say this because more than 80% of participants in a recent CDO Magazine survey of senior data leaders considered leveraging AI a high priority for the C-Suite of their organizations. The participants listed product/offering innovation, improving decision-making, reducing expenditure, and quality work as some of the motivations behind AI adoption.
On the other hand, our research also revealed that the path to AI adoption was faced with multiple critical hurdles like limited skills/talent; data availability, accessibility, and quality issues; confusion over AI scope and use cases, data privacy and security; and transparency and ethical use.
Our findings are in line with the outcome of a recent industry report comprising inputs from 600+ data leaders by Informatica, a market-leading cloud data management company. The report suggests that nearly 100% of data leaders globally who are adopting or planning to adopt generative AI encounter challenges around data quality and privacy.
This scenario circles back to the reality of the current state of data management and how the trustworthiness of data directly impacts the reliability of AI initiatives – Garbage In = Garbage Out.
Not paying adequate attention to this relationship can create added risks during the productionizing of AI models and flow further downstream when leveraging them for decision-making – leading to anxiety and distrust in the AI initiative among users.
During a research-focused interview for CDO Magazine, Mark Mullins, Chief Data Officer at United Community Bank, stated that ensuring proper data management enables data science teams to proactively leverage AI tools, which in turn, will play a key role in areas such as risk avoidance and automation, helping his bank achieve a favorable efficiency ratio (expenses: revenue).
Reliable data fuels confident decision-making and empowers AI algorithms to deliver accurate insights and results. As data leaders across the world embark on the journey towards AI readiness, it is imperative to scrutinize the foundation upon which the intelligent systems are built – the data they rely on.
Trust in data – its reliability and relevance – has emerged as a non-negotiable prerequisite for the effective deployment of AI technologies. Unreliable or inaccurate data can lead to flawed analyses, eroding trust and undermining the utility of AI-driven solutions.
In a landscape where data-driven organizations expect a significant spike in their volume of data – structured and unstructured – and from multiple different streams, achieving trusted data is no small feat. It necessitates a flawless framework of governance – one that addresses the intricacies of data collection, storage, processing, and dissemination.
Dr. Michael Zimmer, Chief Data Officer at Zurich Insurance says that his organization has well-defined governance with responsibility and ownership for data as well as for AI cases. “Ownership is key to compliance as well as data quality and maintenance of AI cases. Besides that, integration patterns are important to industrialize the roll-out of services.”
Achieving trusted, confident, and reliable AI hinges on robust data and AI governance, which serve as bulwarks against various pitfalls. Bias in AI systems can perpetuate societal inequalities. Hallucinations, or false positives, threaten AI integrity. Regulatory scrutiny, driven by data privacy regulations such as GDPR and CCPA, necessitates adherence to stringent governance protocols to avoid regulatory action and reputational damage.
Speaking on the responsible adoption of technologies including generative AI during an interview for CDO Magazine, Andrea Pezzetta, Chief Data Officer at global biopharmaceutical company Ipsen, highlighted privacy and security as potential challenges. She mentioned that her organization is working to put proper guardrails in place, and suggested that companies across the board must lay the framework regarding what good data should look like with generative AI.
Shedding light on hallucinations, she stated that clean data is a must to limit hallucinations and stressed the need to ensure that the training data is clean, accurate, and unbiased.
Historically, data and AI governance have been marred by complexity, hindered by siloed systems and disparate standards. However, the urgency of the AI-driven future demands a paradigm shift. Enter modern cloud-native integrated tools – the catalysts for simplifying the adoption of data and AI governance.
Pezzetta wishes to leverage AI to clean data and look for anomalies. By leveraging a modernized solution approach, organizations can streamline governance processes, breaking down silos and harmonizing standards across disparate datasets. These tools offer scalability, flexibility, and interoperability, empowering stakeholders to navigate the complexities of data and AI governance with ease.
Zimmer too points out that AI is a lens that can help discover data quality issues surely and quickly. More importantly, he says that AI presents a value that further highlights the topic and helps invest in data quality.
“We need to bring AI into our processes. Therefore, we need to define governance processes to develop AI and data together with hubs in business on centralized platforms with integration patterns. I would love to get AI functions in ETL (extract, transform, and load) processes. I hope that we start to use AI in the data pipelines to enhance data quality,” Zimmer adds.
AI holds immense promise in maximizing the potential of data, yet bringing this promise to fruition entails data leaders skillfully balancing multiple priorities and surmounting various challenges.
A whopping 99% of the 600 data leaders participating in Informatica’s survey cited data- or tech-related obstacles to realizing their data strategy. Not only do they grapple with an increasing volume and variety of data they also have to deal with an ever-increasing number of data consumers. Around 41% already struggle with 1,000+ data sources and 79% expect that number to increase in 2024.
Additionally, 30% cite being unable to scale data delivery when and where needed, highlighting that it is not just the data, but the consumer, that is making data leaders’ jobs more difficult. Other priorities include improving data privacy and security (36%), as well as driving more automation in data management and governance processes (34%).
Speaking of data management, 34% recognize their lack of a complete view and understanding of their data estate, with many realizing it is too fractured: 33% cite data or application silos and fragmentation among their top obstacles.
Multiple data leaders have mentioned these issues during their interviews for CDO Magazine. They also mentioned other challenges such as tool sprawl, legacy systems, and inadequate investments.
Informatica’s study also revealed that data leaders face a host of challenges unrelated to the data or technology. They are more related to issues around change, literacy, leadership, and organizational culture. Around 98% admitted to organizational obstacles holding back their data strategy. The problems include a lack of leadership support and sponsorship; an inability to justify ROI for data investments, and a lack of cooperation/alignment across business units.
While Informatica’s detailed survey provides a more structured overview of the scenario, these insights have repeatedly surfaced across CDO Magazine’s interviews with data leaders from across industries over the last several months.
In one such interview, Sravan Kasarla, Chief Data Officer at not-for-profit financial services organization Thrivent, stated that increasing the “data IQ” of the organization as a whole is critical for the success of its data strategy.
In terms of ROI and data investments, Hong Gao, Executive Director of Enterprise Data and Analytics at Indiana Farm Bureau Insurance, stated in her interview that the data unit of any organization should not be seen simply as a cost center but something that can be used to generate revenue. She mentioned that her team works with business partners to drive value by understanding their needs and helping them work faster and easier.
On the issue of support and sponsorship, Ioana Mazare, VP of Enterprise Data Strategy at UPS, mentioned that leadership support is fundamental for success, with business leaders becoming advocates for data-driven mindsets, championing data and analytics initiatives within their functions.
A CDO’s list of AI challenges has a significant “People” factor too. The Data and Analytics industry has been facing a talent shortage, especially in the AI and ML space for years now. Over that, the increased pressure of delivering AI is proving to be a thorn in the side for data leaders across industry sectors.
There is a critical need to address the talent gap and promote literacy in data science and AI technologies to fully leverage the potential of AI. Additionally, the adoption of automated tools and unified platforms plays a pivotal role in facilitating upskilling and reskilling efforts while simplifying access and scalability.
Around 60% of participants in CDO Magazine’s survey cited limited skills and resources as a barrier to AI success. This has led to leaders exploring multiple approaches to solve the problem including exploring external partnerships to upskill internal talent pools, mingling data with business teams, improving data access with self-service analytics, adopting the latest tools and data capabilities; allowing paid learning hours, and initiating upskilling/reskilling programs and communities.
Unsurprisingly, almost half of the participants in Informatica’s survey were considering upskilling or reskilling their staff on AI and machine learning. The skilling efforts also included other areas of interest including data analytics and visualization; data engineering, data governance, enterprise architecture, and DataOps.
The convergence of AI and data management holds immense promise for organizations seeking to leverage their data assets effectively while ensuring compliance, security, and agility. One of the key advantages of integrating AI in data management lies in its ability to automate governance processes for greater reliability.
In an opinion piece exclusively penned for CDO Magazine, Amitabh Seli, CDO and Head of Data and Analytics at Danone (UK), highlighted the pressing need for data leadership teams to harness the power of AI as it advances and creates new possibilities in data and analytics in 2024.
Seli predicted low-to-moderate adoption/use of generative AI in data teams. “Generative AI will be increasingly used to convert old programming scripts to current languages during platform migrations thus delivering trust and security.”
He also highlighted the emergence of AI’s application in master data management (MDM) and data governance. “This will have a very strong and positive impact on the projects worked on by the analytics teams. Data leaders must however remain cautious and focused to ensure AI-based decisions remain unbiased and transparent.”
“The rapid advancement will continue into the next few years and bring with it an additional challenge for the data teams to stay abreast of changes and developments in the field at large,” Seli added.
AI-powered data management tools can learn from data patterns and user interactions, and adapt to evolving business needs and regulatory requirements seamlessly. This agility enables organizations to respond swiftly to market changes, while still upholding data integrity and security standards.
Several use cases demonstrate the potential of trusted data as the foundation for trusted AI, yielding benefits across various domains. For instance, in compliance-driven fields such as Environmental, Social, and Governance (ESG) reporting, AI-powered data management can facilitate the collection, analysis, and validation of relevant metrics, ensuring accuracy and transparency in reporting processes.
Additionally, the migration of data to the cloud is made safer and more efficient through AI-driven data discovery mechanisms, which identify sensitive data elements and assess their compatibility with cloud environments with greater speed and accuracy over manual efforts.
Furthermore, AI-powered data management enables trusted access to data stored in data lakes and data warehouses, enabling organizations to derive actionable insights while maintaining data privacy and security protocols.
Latha Subramanian, Senior Vice President and Global Head of Data Analytics at GM Financial, points out that technologies like generative AI can play a significant role in scaling data governance. “Companies can derive large-scale process efficiencies as they can quickly derive information from complex data sets and sources to curate information and insights on new policies, etc. As a result, the data stewards and data owners can easily leverage data for rapid decision-making.”
She further adds that AI can be used effectively to drive faster decision-making in data quality, reflecting business glossary updates. “AI can not only be limited to data management but also to the data integration lifecycle for quality assurance, development, and other facets of a data migration journey. It helps shorten the development lifecycle and delivers process efficiencies to the IT organization.”
By mitigating the risk of data-sharing between producers, consumers, and data products, AI-powered data management fosters a culture of trust and collaboration within and across organizations. This leads to accelerated time-to-value, streamlined collaboration processes, and a unified user experience through intuitive interfaces.
On the AI talent front, Wendy Lawhead, Director, Analytics Engineering - BiopharmaDirector/TL, Analytics Engineering – Biopharma, at Pfizer, pointed out in a CDO Magazine Interview that the development and retention of AI talent also depended on ensuring they have the tools to help them be successful in their roles and add value to the business.
Lawhead noted that data science talent is expensive and not providing them with the tools and the data to be successful in their jobs can lead to wastage of resources. (Lawhead was Director of Enterprise Analytics at McKesson at the time of the interview.)
On a similar note, Subramanian mentions that being on “scalable, trusted, and extensible cloud data platforms is one of the best-in-class technology trends” that analytics teams are leaning towards to ensure that they can quickly get insights into their corporate data. “Most companies sink millions of dollars into their AI Practice only to realize that the data is yielding inaccurate business insights,” she concludes.
Amidst the swift advancement of AI and analytics capabilities, data organizations are under immense pressure to leverage diverse AI applications across industries, akin to an intense "AI Arms Race" among businesses where market competitiveness is key. Efficiency has historically proven essential for navigating market dynamics effectively and assuming industry leadership.
However, achieving AI success comes with significant hurdles, including remediating data quality issues, solving talent shortages, and concerns surrounding privacy and security exposure. Moreover, there's a growing focus on the ethical implications of AI implementation, reflecting heightened societal concerns.
Data leaders recognize that AI readiness hinges on accelerating data readiness through automation, particularly in areas such as data governance and ETL processes. AI-powered data management tools are seen as essential for enhancing data reliability and streamlining processes, considering manual tasks can be both time-consuming and error-prone.
Furthermore, it's imperative for data offices to enable AI talent to continuously enhance their skills and capabilities, leveraging tools, simplifying collaboration, and supporting external partnerships to thrive in their roles.
Data and AI leaders featured in CDO Magazine express enthusiasm and acknowledge the challenges ahead, including bolstering data quality, privacy, and integration, in preparing for AI. Prioritizing data readiness is crucial to them and they are open to exploring integrated solutions that facilitate the seamless utilization of trustworthy data by consumers and for use cases, ultimately aiming for trustworthy AI implementation.
Informatica (NYSE: INFA) brings data and AI to life by empowering businesses to realize the transformative power of their most critical assets. When properly unlocked, data becomes a living and trusted resource that is democratized across your organization, turning chaos into clarity. Through the Informatica Intelligent Data Management Cloud™, companies are breathing life into their data to drive bigger ideas, create improved processes, and reduce costs. Powered by CLAIRE®, Informatica's AI engine, it’s the only cloud dedicated to managing data of any type, pattern, complexity, or workload across any location — all on a single platform. Informatica. Where data and AI come to life.