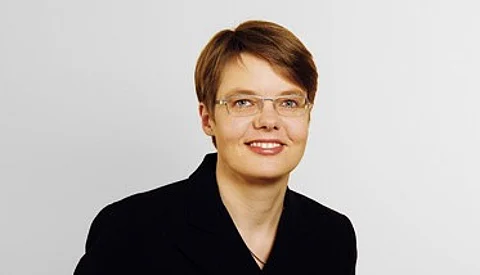
With the exponential growth of data, the challenges surrounding data quality are also on the rise. Once a year, the Competence Center Corporate Data Quality (CC CDQ), together with the European Foundation for Quality Management (EFQM), honors companies with outstanding data management initiatives that address data quality challenges.
The three finalists Merck, Nestlé, and SAP demonstrated innovative approaches, laying the foundation for exploiting the value of data — in operational business processes, data-based decisions, or new business models.
Learnings from Merck – Identifying critical errors with meaningful data quality scores
The principle "You can't manage and improve what you can't measure," also applies to data quality. However, even companies that measure data quality do not necessarily see improvements.
Pharmaceutical company Merck (MSD – Merck, Sharp & Dohme) tackled this challenge by demonstrating how data quality can be measured in a way that identifies critical errors and sets the right priorities for data quality improvements.
For Merck, the quality of its product master data is business-critical for digital transformation, specifically in supply chain management. However, when a data quality dashboard was introduced as part of the master data program, there was virtually no change in the number of data errors despite the fact that some of the errors were costly and always exceeded the target value of the so-called pass rate (the quotient of passed data records and the total number of data records).
In June 2021, Merck introduced a Data Quality Score as a new measurement method that evaluates and weighs errors according to their individual relevance, taking into account the business relevance of the error (criticality for the business process and the importance of the business segment) and its dynamic aspects (the lead time and the age of the error).
One advantage of the score is that it can be aggregated along the relevant dimensions. For example, per region, business unit, or product, allowing data quality metrics to be presented in user-centric dashboards for different stakeholders.
Compared to the pass rate, the Data Quality Score provides more meaningful information and key performance indicators (KPIs) in terms of the business impact caused by data errors and where to prioritize error elimination. The end-to-end architecture is also noteworthy. Data quality measurements build on a data lake with a data quality engine and a data mart for dashboards and simultaneous integration with the data catalog.
Learnings from Nestlé - “First time right” with automated business rules
Getting key (master) data correct is often time-consuming and requires input from a variety of functional experts. If errors do creep in, corrections are very costly and cause many consequential problems in the business processes. Nestlé's approach to improving data quality in its master data management was to create new data records as error-free as possible - also known as "first time right."
Nestlé's good practice shows how the creation of new material data and its subsequent localization for different countries or plants can be automated to the greatest possible extent by means of certain business rules. At the same time, data quality was significantly improved.
For Nestlé, as a consumer goods producer, direct materials are among the most critical data in the company, representing raw and packaging materials as well as semi-finished and finished products. Quality and timeliness of material data are key to effective business processes in product development (Idea-to-Product), procurement (Procure-to-Pay), production (Plan-to-Execute), order processing (Order-to-Cash), and accounting (Record-to-Report).
The creation of material data is a complex and labor-intensive process that ranges between 15 to 30 days and must be repeated for each plant in which the material is used. Globally, this results in several hundred thousand requests for material plants per year.
Not only is this process lengthy, but it also requires the input of numerous functional experts who must understand the details of the various material fields to enter the correct value for a given context, resulting in data quality issues that negatively impact process efficiency.
Nestlé's idea was to automate the entry of material fields as much as possible using predefined business rules to ensure a consistent, fast, and transparent material activation process – even in times of organizational change. In a global project, the commonalities, patterns, and potential for improvement in the material system were first analyzed by 600 experts worldwide. The business rules were then defined and implemented using a standard tool: the SAP Business Rule Framework.
Through automated business rules, Nestlé achieves high-quality and consistent data and functional experts are relieved of administrative burdens. New materials can now be activated in seconds or days instead of weeks or months. The solution has proven scalable, with the global coverage already having reached 80%.
Learnings from SAP - Machine learning to extract master data from free text data
Forms are highly popular in online campaigns to collect information from prospects and customers. Since all information cannot be directly captured in a structured way, free text entries are often utilized to solve the problem. To subsequently process this data in an automated way, it is often necessary to manually rework the entries and record them in customer relationship management (CRM) and other systems.
SAP's good practice shows how machine learning methods can be used to extract structured master data directly from free text entries. The starting point was a backlog of more than two million contact details from various forms in which job titles and department names of contacts were recorded as free text fields.
With the existing mapping tables, only about 50% of the recorded information could be transferred directly into the CRM system, so employees had to post-process it manually. The considerable effort needed due to the different contexts, languages, and millions of different job titles caused a backlog that was no longer manageable.
Using machine learning, SAP implemented a scalable data mapping process. With a classification process, the free text information captured from the customer is converted into standardized codes with information on position and department, which can then be used for marketing and sales purposes.
The approach not only increased the degree of automation to 80%, reducing manual mapping work drastically but also processed the forms much faster. Since contact information ages quickly, the process thus also improves the success rate of marketing campaigns and sales activities.
SAP was able to capture the business value in the different phases of the project, ensuring end-user acceptance and integration with the existing tool and architecture. Based on the compelling experience in extracting structured information from free-text fields, SAP plans to expand the use of machine learning in data management.
Key takeaways from the three cases:
The needs for high-quality data are indisputable but highly challenging to address. The winners of the CDQ Good Practice Award this year have demonstrated three innovative methods that can significantly improve data quality:
Meaningful data quality scoring that helps prioritize and eliminate errors where they are most problematic
Business rules to automate master data creation (first time right)
The use of machine learning methods to extract high-quality master data from free text entries
About the Competence Center Corporate Data Quality (CC CDQ):
The Competence Center Corporate Data Quality (CC CDQ) is a unique industry-research consortium and Europe's leading expert community for data management. Members of the CC CDQ benefit from a cross-industry network and knowledge sharing, in addition to cutting-edge research and co-innovation.
The CC CDQ connects data management and analytics experts from practice and academia comprising more than 20 renowned Fortune 500 companies and multinationals stemming from a variety of sectors. The research team is located at the Faculty of Business and Economics (HEC) of the University of Lausanne and is headed by Prof. Dr. Christine Legner, who co-founded the center in 2006 at the Institute of Information Management (IWI – University of St. Gallen). Today, it is operated by CDQ AG.
Author Christine Legner is a professor and director of the Department of Information Systems at the Faculty of Business and Economics (HEC), University of Lausanne, Switzerland. She is also the head of the Competence Center Corporate Data Quality (CC CDQ), a research consortium and expert community in the field of data management.
In this role, she closely collaborates with leading European companies to support them in managing data as an asset. Before joining HEC Lausanne, she was a professor at European Business School and a postdoc at the University of St. Gallen. She was also a visiting scholar at INSEAD and Stanford University.