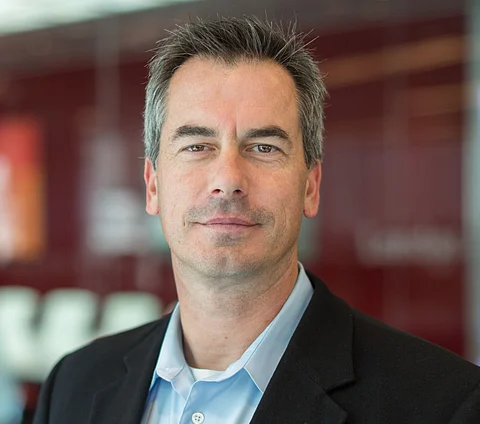
The modern data profession is still young and has been constantly innovating since it began roughly 20 years ago. At the end of the 90’s and into the early 2000’s, there was a subtle shift toward treating data as something in its own right, as opposed to stuff to be stored, sent in messages or wrangled in some ETL process—thus kicking off the data profession. As with any innovation, there were false starts, dead ends, and a vast amount of learning. Through these growing pains, brilliant new methodologies, technologies, and new ways to apply old methodologies began to show how we, as data practitioners, can inform and add value to the industries in which we work.
One of the ways data practitioners can contribute is to solve context and semantic problems in data by employing ontology to create formal models and relationships, based around utilizing triples and leveraging RDF and OWL as modeling techniques. These are, of course, concepts borrowed from philosophy and linguistics but have taken on their own form, losing connection to those disciplines. This can be seen in the effective ‘rebranding’ of ontologies in information and data sciences as “knowledge graphs.”
In this embracing of knowledge graphs and ontologies for data, the linguistic and philosophic underpinnings have mostly gone ignored. Technology, based in math and science has precise answers, and in industry, tools to solve data has always been technology-forward. Linguistics is much more of an art than science, and in the majority of data science programs is given only passing mention, if at all. As data grew from the technology solutions of SQL and Oracle databases, technology continued to be the focus to find answers.
The focus on triples brings out the argument that we should all concentrate on the ‘atomic elements’ and be as granular as possible, therefore removing any nuance or possibility of misinterpretation. This fuels a viewpoint, drive, and belief in a ‘single version of truth’, as if we can all just agree on a single financial language for all. This false narrative implies that by removing context at the lowest levels, and by just looking at “the data”, somehow context and complexity goes away. But there is very little to be gained from an overly granular, data-point-by-point approach since it loses the bigger picture.
This is not to say that ontologies or knowledge graphs are not useful. If anything, they are critical tools but must be used properly, which is where the domain comes in. There is a general acceptance for needing ‘domain ontologies’ and ‘upper ontologies’ but what these signify and what qualifies as a ‘domain’ is very much left undefined and open. As data professionals, this lack of specificity or any methodology should raise flags.
Within linguistics, and more specifically applied linguistics, there is the concept of language-specific communities such as ‘speech communities’, ‘communities of practice’ or ‘discourse community’. In my book, “Understanding the Financial Industry through Linguistics,” I explore the use of Communities of Practice (CoPs) to better define ‘domains’ within financial services to enhance the usage of ontologies and knowledge graphs.
The challenge in defining the scope of a CoP is that it needs to be specific enough to bound a shared language, but wide enough that it is not limited to a completely isolated population where linguistic variability is deminimus. For example, the financial services industry as a whole is vastly too broad, but defining it as five people on a trading desk at firm X is too specific to be a meaningful scope for a CoP.
Based on my research, I define how to bound a CoP using Wegner-Treynor’s (among others) elements of a “domain,” a “community” and a “practice.” A domain is a shared function, where learning is shared. A community is the bond through learning, either culture or shared goals, for the function. And the practice is processes that are used within that culture (community) to perform the functional goals (domain). One of these things alone do not do the whole job in defining a CoP, as you can have two different communities performing the same processes but for different functions (domains), and should rightly be viewed and treated differently in regards to their language, culture, and data.
CoPs are the parts that make up a larger social system. For me, that social system is financial services. Each CoP (part) does not survive on its own, nor are they necessarily mutually exclusive. Although they have their individual functions and goals, they are reliant on the overall health of the larger social system to continue to operate.
CoPs do not infer homogeneity, but they do lend themselves to having their own unique shared language – inclusive of jargon – and that language is embedded in the data they store and generate. It influences the data they receive from other outside CoPs and influences how they interpret, use, and store that data. Such that the ‘same’ data received from CoP ‘A’ can actually differ in meaning and inference when CoP ‘B’ consumes and stores CoP A’s data. The consuming CoP B is interpreting, and therefore potentially changing, the meaning of the data through the simple process of consumption.
Here we can look back at the discussion on defining ‘atomic elements.’ Regardless what you call them, they are data. And data is language stored, in context, by those storing it. Chomsky spoke of a ‘universal grammar’ which is akin to this atomic element concept. Essentially this is the idea that you can find ‘common meaning’ on very basic concepts. But while we can all agree what a ‘nose’ is, that common meaning becomes irrelevant in the face of the variability of noses. As far as atomic elements, we should remember atoms are composed of smaller elements, and those parts can even be further reduced.
So, if going as granular as possible is not a realistic solution, and a single financial language that everyone can agree upon is linguistically impossible, how do we even start the process of using graphs and ontologies without boiling the ocean?
This is where there will likely be some friction. Applied linguistics is much more of an art than a hard and fast ‘black and white’ solution that technologists are used to. As data professionals, we want a definitive answer – ambiguity presents all sorts of issues. But attempting to ignore that ambiguity exists, does not make it go away.
As expressed in the Communities of Practice Matrix image, and explained previously, CoPs are not mutually exclusive. One individual can belong to more than one CoP: overlap occurs at interaction points, as well as overlap due to horizontal versus vertical processes and functions. As a result, defining, scoping, and bounding CoPs ends up being much more ‘art’ and subjective rather than something with firmly set boundaries in perpetuity.
Formally including a CoP analysis before delving into data analysis and definition exercises to populate knowledge graphs greatly helps to streamline processes later on. When new objects come in, these should first be qualified on which Cop they belong to as well as how seemingly the ‘same’ objects may actually differ depending on the CoP. This process lends to better direction on when, where, and how to create linkages between graphs and if that link should or can be made at all. It also provides a mechanism to manage the translation and the interoperability of connected graphs over time, understanding that a change in one graph may result in a need to re-examine the translation and connection to a graph belonging to another CoP.
Today, data practitioners create models based on the immediate world around them. Sometimes these models partially relate to existing CoPs or silos, but they lack formality, don’t account for potential conflicts or don’t interact with models outside their world. Formally including a CoP analysis at the front end of any data activity when a new graph is encountered, creates a starting point for commonality in methodology. Determining which CoP that new graph applies to is a key exercise.
Within an enterprise, does the data cross front, mid and back office? Each of these are different CoPs with different languages (different jargon), and different data needs based on their function and processes. Is the project crossing equity and fixed income? Again, formally separating these CoPs, in regards to the data exercise and then translating between the two – rather than trying to merge and find lowest common denominators.
This provides the basis for understanding how, and if, graphs may interact, and how much translation work is required, offering possible starting points for interoperability and linking.
copyright; Robinson, Richard C. “Understanding the Financial Industry Through Linguistics”; Business Expert Press, 2021.