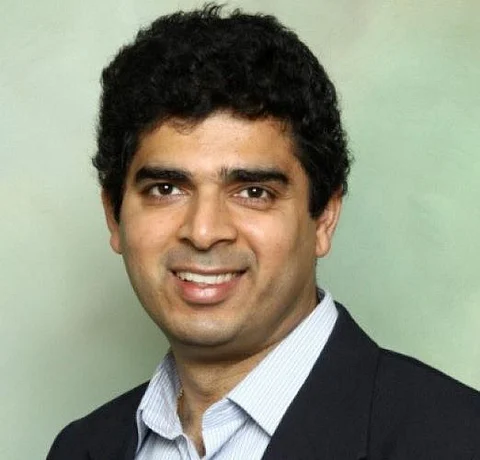
The amount of data generated by the businesses today is unprecedented. According to the World Economic Forum (WEF), by 2025, it is estimated that 463 exabytes of data will be created globally each day — that’s the equivalent of 200 million DVDs per day [WEF, 2019]. Why is so much captured and processed? Data has enabled firms such as Netflix, Facebook, Google and Uber to have a distinct competitive advantage. In 2021, the market capitalization of Amazon ($1.7 trillion) was more than the combined GDP of two big G20 countries — Turkey ($780 billion) and Saudi Arabia ($700 billion). Fundamentally, companies that are data-driven demonstrate improved business performance. A report from MIT says digitally mature firms are 26% more profitable than their peers. A McKinsey study found that data-driven organizations are 23 times more likely to acquire customers, six times as likely to retain customers, and become 19 times more profitable. Overall, data today is seen as the next frontier for innovation and productivity in business for a sustainable competitive advantage [Southekal1, 2020].
However, according to Experian Data Quality, a boutique data management company, poor quality of data affects the bottom line of 88% of organizations and impacts up to 12% of revenues [Experian, 2015]. A research study published in Harvard Business Review (HBR) says that just 3% of the data in a business enterprise meets quality standards. Joint research by Carnegie Mellon University and IBM found that about 90% of the data is never used for business activities [Southekal2, 2020]. All this research points to the fact that data quality is low in businesses today and needs to be fixed. One solution to improve the data quality in business is with effective data governance.
What exactly is data governance? According to Gartner, “Data governance is the specification of decision rights and an accountability framework to ensure the appropriate behavior in the valuation, creation, consumption and control of data and analytics.” [Gartner, 2022]. So, why is data governance needed? The main purpose of data governance is to securely manage the quality of data in the entire data lifecycle — from capture to consumption — so that the right people are managing the right data in the right manner. An effective data governance program addresses three key questions or elements:
What data to govern
How to govern data
What organization mechanisms are required to govern data
Let us start with the first element in data governance — what data objects to govern? In data governance, data comes before governance. Given that there are various types of data in a business enterprise with varying degrees of importance, the selection of data objects should be based on business value. In this backdrop. there are many types of data assets in a business enterprise:
Reference data on business categories like plants, account groups, payment terms, shipment priorities, and so on.
Master data for business entities like customers, vendors, products, GL accounts and more.
Transactional data on the business events like orders, prices, invoices and so on.
While the specific data type to govern depends on the industry sector and the prevailing business need, data governance works effectively on data objects that are:
Managed early in the data lifecycle especially during data capture and data integration.
Shared and reused enterprise-wide in business transactions like purchase orders, sales orders, and invoices.
Fundamentally, if high data quality is desired, then the data governance practices should focus on reference data and master data in the initial stages of the data lifecycle (DLC). A simplified example of a data flow diagram (for both structured and unstructured data) in a business enterprise with focus on data governance is shown in the figure below.
Figure 1: Data Governance in the Simplified Date Lifecycle (DLC)
The second element of data governance is how to govern data. This is mainly about setting up the 3Ps — Policy, Process and Procedures.
A policy is a rule that helps an organization govern the data and manage risks based on standards. A standard — internal or external — makes the policy more meaningful and effective. Sample data standards include naming standards, taxanomy, data modeling standards, data architecture standards, and so on.
A business process is a series of related, structured activities performed by the data governance team to accomplish a specific objective. These processes could be on data quality surveillance, data exchange, data lineage tracking, data profiling, validation of compliance to regulations, data archiving, and more,
A procedure is a sequence of steps or work instructions to complete an activity within a process. For example, the data archiving procedures could be inventorying and determining which data must be archived, assigning a retention schedule for each data object, and so on.
Figure 2: Policy, Process and Procedure Hierarchy
Finally, data governance is not just about setting policies, processes, and procedures for data. At the core, data governance is a cross-functional activity. Hence, the third element of data governance is setting up organizational mechanisms to govern data. Based on the work on Gregory Vial [Vial, 2019], the three organizational mechanisms to govern business data are structural, procedural and relational:
Structural mechanisms are about the creation of data governance roles to enable the creation of policies, processes and procedures. A good data governance program typically includes the steering committee with three main groups: data owners, data stewards, and data custodians. The three positions all work together to create the policies, process, and procedures for governing data, especially the reference data and master data elements.
The data owner, who is from the business, is accountable for the data and makes decisions on the right to access and usage.
Data stewards are from the various business units, and they are responsible for the content and context associated with the data.
Data custodians are from IT and they are responsible for the safe and secure custody, integration, and storage of data.
Procedural mechanisms are used by the organization to ensure compliance to the structural mechanisms. This is where the data owner, the data stewards and the data custodians come together to monitor data quality with appropriate data profiling KPIs. Specifically, data quality monitoring includes setting specification limits, thresholds, and targets, ensuring conformance to those values, and effectively communicating the KPIs to stakeholders for taking corrective measures.
Relational mechanisms include key activities to support collaboration between different data governance teams. Effective data governance requires data owners, data stewards, and data custodians to jointly take complete responsibility for the quality of the data in the enterprise. The data stewards and data custodians who are responsible for data quality work under the strategic direction of the data owner who is accountable for the quality of the data object.
Figure 3: Data Governance on Customer Master
These three elements, i.e., data objects, 3Ps, and organizational mechanisms, can be implemented with data governance tools from leading companies such as SAP, Informatica, IBM, Collibra, Alation, and more. These data governance programs offer regulatory compliance, enhanced privacy and security, data classification, and more while enabling organizations to access, curate, categorize, and share data wherever they reside.
A good data governance program with the three elements discussed above can ensure high data quality as it brings focused execution based on the strategy. Today, data governance is not an option; it is a required capability if the enterprise wants to use data for improved operations, compliance, and decision making. Data governance also helps in risk mitigation because businesses today hold incredible amounts of data about customers, suppliers, prices, products, employees, and more that must comply with laws, regulations, industry standards, internal business processes, and ethics. Overall, data governance helps businesses to properly and proactively manage data and reduce its financial and compliance liability. This means good quality data, better models, better insights, better business decisions, and ultimately superior business performance and results.
References
Experian, “Is Dirty Data Costing you?”, https://www.xperience-group.com/the-cost-of-dirty-data/, 2015
Gartner, “Data Governance”, https://www.gartner.com/en/information-technology/glossary/data-governance, 2022
Southekal1, Prashanth, “Analytics Best Practices”, Technics Publications, April 2020
Southekal2, Prashanth, "Illuminating Dark Data in Enterprises", https://www.forbes.com/sites/forbestechcouncil/2020/09/25/illuminating-dark-data-in-enterprises/?sh=37e4fd6bc36a, Sept, 2020
Vial, Gregory, "Data Governance in the 21st-Century Organization," MIT Sloan Management Review, Oct, 2020
WEF, “How much data is generated each day?”, https://www.weforum.org/agenda/2019/04/how-much-data-is-generated-each-day-cf4bddf29f/, Apr, 2019
About the Author
Prashanth Southekal is the Managing Principal of DBP-Institute (www.dbp-institute.com), a Data and Analytics consulting and education firm. He has consulted for over 75 organizations including P&G, GE, Shell, Apple, and SAP. Dr. Southekal is the author of two books — “Data for Business Performance and Analytics Best Practices”, and writes regularly on Data, Analytics, and Machine Learning for Forbes.com, FP&A Trends, and CFO University. Apart from his consulting pursuits, he has trained over 3,000 professionals world-wide in Data and Analytics. Dr. Southekal is also an Adjunct Professor of Data Analytics at IE Business School (Madrid, Spain) and an Instructor with TDWI. He holds a Ph.D. from ESC Lille (FR) and an MBA from Kellogg School of Management (U.S.). He lives in Calgary, Canada with his wife, two children, and a high-energy Goldendoodle. Outside of work, he loves juggling and cricket.