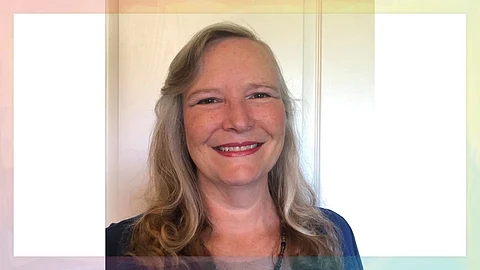
A novel approach to handling complex interconnected IT systems known as AIOps (artificial intelligence for IT operations) applies artificial intelligence (AI) to help automate many IT tasks. This includes analyzing large data sets to identify problems before they escalate to the point where customers become aware of an issue.
This approach harnesses the power of AI and machine learning (ML) to manage the performance and reliability of IT hardware, software, and applications. It helps in detecting anomalies, adapting to changes in requirements, responding to incidents, and proactively adjusting systems to minimize disruption of services.
The core value of AIOps lies in its ability to automate routine and repetitive tasks such as log analysis, event correlation, and incident triaging. On the front lines, IT teams receive a high volume of alerts from various systems and tools.
Alert fatigue can make it challenging to identify critical issues promptly. AIOps helps reduce alert fatigue by reducing noise, prioritizing alerts, and providing actionable insights into root causes.
AIOps can help by not just identifying problems, but pointing at the clues in the data that indicate what caused them or will cause harm in the future. By catching issues early, IT teams can reduce downtime and improve the availability of services.
AIOps helps to:
Analyze historical data and usage patterns to enable organizations to gain deeper insights into their infrastructure
Monitor current data and usage, compare that to past patterns, and flag when bottlenecks, component failures, or other issues might be occurring
Predict future trends to optimize resource allocation, and plan for future growth. (E.g., capacity planning and change impact analysis)
One obvious benefit of AI is that it saves significant costs and time over more manual methods of IT operations management
A good example of AIOps would be the analysis of traffic in 5G telecom networks to identify overloaded areas, and re-route data to less burdened towers. Another would be the analysis of hardware monitoring data to spot trouble areas and identify the specific lines that point to the possible root cause from the mountain of log data.
Predictive maintenance is another excellent use case for AIOps. By analyzing past usage and failure patterns, ML models can identify similar patterns in current data that indicate an equipment failure about to happen, in time to repair it before it fails.
Although AIOps projects hold tremendous potential for organizations to achieve breakthroughs in IT operations management, studies show that only about half (53%) of AI projects accomplish the move from prototype to production. Several factors add to that difficulty:
1. Increasing complexity in IT
The more complex an architecture is, the more difficult IT monitoring and optimization projects become. Legacy systems and data silos lead to fragmented and conflicting views of the system.
Integrating real-time streaming and historical data sources as well as integrating many distinct types of data, semi-structured log, trace, and event data with structured data and correlating with KPIs is often the solution.
AIOps helps correlate and contextualize that vast array of data to make it useful.
2. Massive data volumes overwhelm systems
The average enterprise streams data from about 135,000 endpoint devices along with hundreds of Internet of Things (IoT) devices that are cropping up everywhere. A growing sprawl of enterprise applications generates even more data.
Proactively detecting abnormalities, and predicting or resolving issues requires AIOps to store each instance of data (not data aggregations). Most clues to network issues are hidden at that fine granularity.
As data volumes expand, accessing, analyzing, querying, correlating, joining, and processing data at this fine-grained level becomes more of a challenge.
3. Setting up good, continuous data flows
Data is the heart of AIOps. AI algorithms need access to a large amount of historical data. This teaches the system to understand what normal operations look like and what issues tend to look like.
There is a need to analyze historical data in-place since moving that amount of data is unwieldy and slow. Next, AIOps needs constant, rapid access to current data as it streams in. This allows the application to recognize when current conditions do not match normal conditions, indicating that something is wrong.
A full-scale AI deployment continuously collects, cleans, transforms, labels, stores, and analyzes large volumes of data. IT teams sometimes fail in scaling AI projects beyond test beds and into full production because they lack the tools to create and manage a production-grade data pipeline.
4. Deploying the right data platform is key to getting your AIOps right
Despite the hurdles, AIOps is the future and there is a lot to be optimistic about. The savings in time of IT experts alone are huge, but the improvement in customer service levels is what tends to make the biggest impact.
That said, it is advisable to establish a solid data foundation first before jumping on the AIOps bandwagon. Below are some key considerations for that data foundation:
1. Analyze all existing data
Do not just take samples or settle for siloed views. Deploy a platform that allows you to analyze your entire dataset at low granularity, so you do not miss anomalies.
This is completely attainable and reasonably affordable with modern technology like exabyte scale databases and data lake query engines, and it is essential for root cause analysis and similar AIOps use cases.
2. Analyze streaming data in real time
Data from devices streams in constantly. Your platform should be able to rapidly load data in parallel, query it in time windows, and get fast answers, regardless of where the data resides or what format the data started in.
3. Future-proof analytics
Consider how IT operations evolve as new technologies are introduced or internal dynamics change. As data volumes and numbers of concurrent users or workloads inevitably grow, a solid data foundation should be able to scale to keep up.
As conditions change, the data foundation should also be able to change. Flexibility in deployment options, for instance, keeps organizations from being locked into one that no longer meets their needs.
Whether you are building AIOps to sell to other organizations or deploying AIOps to improve your own organization’s IT operations, the goal is to make the people involved in IT operations that much more effective. AI plus human is more powerful than either human or AI alone.
About the author:
Paige Roberts is Open Source Relations Manager for Vertica by OpenText, a scalable analytical database with a broad set of analytical capabilities and end-to-end in-database machine learning. With over 25 years of experience in data management and analytics, Roberts has worked as an engineer, trainer, support technician, technical writer, marketer, product manager, and consultant.
She contributed to “97 Things Every Data Engineer Should Know,” and co-authored “Accelerate Machine Learning with a Unified Analytics Architecture” both from O’Reilly publishing.