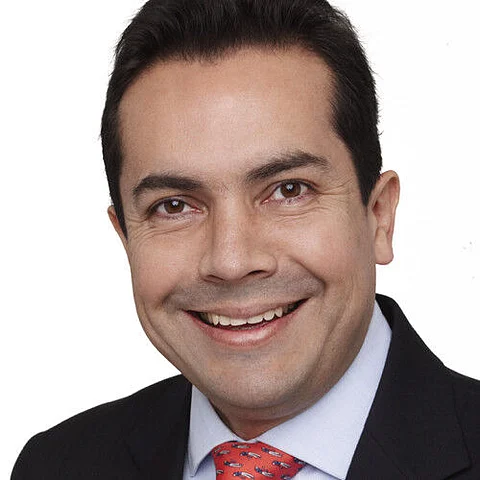
(US and Canada) Luis Alejandro Velandia, Chief Data Officer, Best Doctors Insurance, speaks with Sanjay Acharya, VP Strategy and Growth, HiLabs Inc., about the application of ML and the ideal approach to building in-house data science capabilities.
Over the last several years in the industry, Velandia has participated in practical applications of machine learning and deep learning in multiple industries like banking, health insurance, mining, and retail.
He says that it is necessary to understand the underlying business problem before applying machine learning to decide whether the problem is appropriate for machine learning. Regarding uses, he notes cases involving customer lifetime value, predictive maintenance, branch network optimization, policyholder behavior, predicting illnesses and treatments, binary classification, etc.
“However, the ones that are so compelling are those that include the human element — ML models that can predict healthcare outcomes,” Velandia adds. In one of the previous companies I worked for, we built a few machine learning models to predict if a member is going to be hospitalized in the next 12 months. The insights we could get from the models were used to define and implement care coordination programs. Those who joined the program, statistically speaking, could live longer compared to those with similar attributes who did not join. This, for me, is so compelling and it makes me feel very happy.”
An organization’s decision to either build in-house data science, outsource, or go for a hybrid approach to adapt or bring in AI and ML, depends on the level of data analytics maturity of the organization, he continues.
“If the organization has a robust data illiteracy, data culture, and data operating model in place and they're quantifying the impact of their use cases — and if it has a data management framework and a robust data platform, including a data science environment. that underpin the enablement of the implementation of the specific use cases — I would hire a couple of data scientists initially and start building the data science capabilities in-house,” he says.
But if the organization has no data science capabilities and the data analytics maturity is low, the organization should start with defining and implementing a data analytics strategy.
“I would focus on the data governance domain principle, the data platform, and improve the data culture. I would get a vendor to design and implement the data platform with alignment to specific use cases. The quantification of the business value is super important here. I would get help from those vendors to go through that initial learning path, and hire a data scientist to be part of the journey. After some of the data science capabilities have been built, I would focus on strengthening the internal data science capability by hiring more data scientists and creating a data science program,” he concludes.