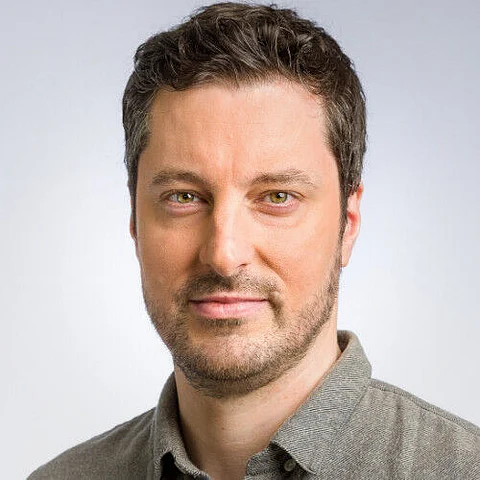
(Europe) Jean-Loup Loyer, Chief Data and Analytics Officer, Eramet, talks with Michael Sieczko, Business Development Director, Lingaro, about the main challenges that he recognizes that enterprise companies have when they try to become more data-driven.
Loyer shares that the first step towards being data-driven is to involve everyone in the vision of becoming more data-driven, and then from the ex-con to the end-users, one really needs to be strong from the very top of the company. So it's a prerequisite because if you don't dive in, then all the layers there probably won't follow. They would just listen and not commit.
If your senior management is not convinced, it is usually challenging to convince. However, the good news is that more and more top managers, CEOs, and top executives are now convinced about the same. So the strong sponsorship is significant, and it's often a given you have or don't. He shares further that middle management is essential also to elbow the initiatives. Usually, middle management is a bottleneck because you need to resolve this in so many places like IT, local businesses, cost control, etc., and the middle management will allocate services. So it's imperative to be able to embark them. And usually, they have so many priorities that they won't help you. For example, suppose you don't convince them that your project will do something meaningful and valuable for them. In that case, they won't help you. Then usually at the end-users, the people at the more operational levels, there is less resistance because they're often interested in having digital solutions to help them. But it doesn't mean that you don't have to convince them.
He shares that once all this is done, it's usually three easy at a personal level. So involving people from the top of the hierarchy to the bottom. When you are putting the right organizational governance in place so that your project is deliverable. So how you organize your work between a business that will be the end-users opportunity to do, the digital, II direction, and directions of the company like methods or engineering. So that's very important and will define primitives and ways to work together on projects. Data analytics is multi-disciplinary by design. So everyone has to start the job well to predict success. So you need someone from my team, someone from the business, from cost control, from engineering expertise, to be sure that it works well.
He shares training is critical if you become autonomous and in charge of the projects. But, there are so many projects to do in the company that you won't be able to do it with a central team, even if it has around 50 people each. It won't be possible until you anchor what you do in the operational reality of your equities on the field. So to do all this, you have to train them and explain them by training. So it is not just a shallow introduction to data management on ethics, but an in-depth training. So we have run projects and 20 programs that last two years and four submitted OGs or geologists. So they code themselves in base camp. They do data science, and they do projects. So we do a lot of project business and be sure that they actually are involved to then use whatever. So that's very important. So I would say training and an appointment is really a self-challenge.
So when you do well on the project, it has a direct impact on the operations. So Loyer sees four main ways for analytics to win a competitive edge for the business. First, analytics can be an excellent way to get a 360-degree view of the business. So, optimizing our operations, minds, and plans by knowing the market price of the competitive solutions and trying to predict what will happen, how the markets will evolve, and how the competition will evolve. He believes businesses are often bred in silos. So thanks to analytics, we can have a good, more factual, clearer view of the business. Second, we have to weigh the competitive edge to accelerate the agility and reactiveness of activity by making faster and better decisions.
So if you freeze the data, for example, and make it accessible to all levels of people across the company, you will help them make money from decisions. In addition, the multiple central decisions in teams and making the decision faster will help you win value over your competitors and reduce conflicts between teams because people will work on shared, clear, and documented numbers.
Thanks to the predictive algorithms, instead of waiting for them to fail, for instance, and then running after all the buns that are failing plants, you can try to predict which is likely to fail in the next two weeks. Then you get to act and be proactive, predict what's going to happen, and be sure that you all are training after you've taught problems.
He shares that through the better optimization of their assets and operations, for instance, reducing the amount of fuel culture by our locomotives, our trucks, which gives us an excellent way to help local communities. We absolutely need the support of local communities around all nines. So we need some win-win agreement in that situation with the corporation and the country we operate in with the project like mining. So he wants to make an environmental impact and social impact. So we can probably improve this relationship and make sure that our company can operate in the long-term.
So for the organizational or human side, there are many engineers, brilliant engineers with quantitative backgrounds and strong expertise in their fields. Usually with good knowledge of physics, chemistry, mechanical engineering, and so on. But ironically, it's an obstacle. It's the beginning of the project. Because these people who have used knowledge and are all very smart and quantitative often don't believe in data-driven analytics because it often lacks the explainability and logic of physics-based models. So often, at the beginning, it can be very tricky to stop a project. But one should convince them by example, through a project that it works and that they have a bit of a picture of their operation, by adding a layer of data-driven analytics. Usually, they understand the nuts and bolts of analytics techniques very profoundly. They become your best friends and allies in this data transformation as they can even switch careers because of the census because everybody has quantitative backgrounds to do so. So it's a very fertile soil to start with, but you need to start the process and convince people.
Our data is often not rooted in many aging proprietaries or nonstandard industrial IoT systems on the technology side. And that makes work much more difficult on both the balance and genetic sides. Because the data goes from Android applications or software, there's the old proprietary. Some of them did back a thousand years, and we have no way to retrench any of them. So it makes things very, very difficult.
So culturally, it's not unique to the case for all the industrial companies that are a bit older. For companies like Google or Facebook or this company will build around data. Data in IT that could be like awesome things for businesses. Sometimes we see a lack of investment or a lot of legacies that throw or put it down.