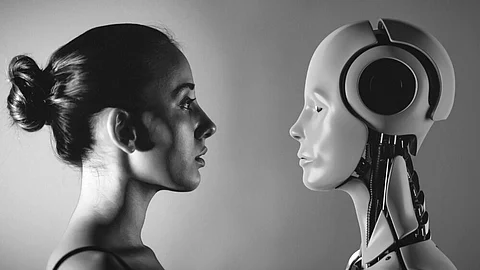
Seventeen years ago, I co-authored an article published in the IBM Systems Journal called Machines in the Conversation. It was about using data mining of informal communication streams to detect themes and trends in human conversation. I argued at the time that whereas the early AI pioneers such as Alan Turing had once envisioned computers and humans having intelligent conversations, the real power of the technology was not in speaking to us, but in helping us better understand what we were saying to each other. I believe this is truer today than ever.
Recently, AI research prototypes such as ChatGPT have captured the public’s imagination and also stoked some fears about where AI technology is heading. I believe that these programs, while valuable in certain narrowly defined roles, are nowhere close to doing some of the high-level creative tasks that they are proposed for. In fact, such efforts misunderstand the true nature of the human-machine partnership. The value of machines to us is not in what they say, but in what they hear…and how they hear it.
To put it bluntly, in most business contexts, the ROI of an ML application is directly proportional to the amount of text it enables us not to read — not the text it enables us not to write. We should fear AI, but not because it might replace us. We should worry that AI may instead steal our lives away, moment by moment, one plausible but misleading answer at a time.
We are momentarily enamored (and maybe a little apprehensive) of the creative, generative possibilities of AI. As a partner in the creative process, AI could indeed help us be far more productive writers, researchers, and even artists. But as a generator of content, all AI can really do is summarize and mimic already existing content. Sometimes this can be impressive, sometimes laughable, but it’s still just a gimmick — a “What” without a “Why.”
The necessity for a more data-centric AI
The proper and most effective use of AI is to effectively analyze content rather than generate it. AI can consume as much content as we care to throw at it, sift through it with endless patience and thoroughness, and come up with the key relevant data that we care about. But we, as savvy AI consumers, need to always take the results that AI presents with a healthy dose of skepticism.
Being able to read more data doesn't always make you smarter, because crowds are not always wise, and the results (at best) are only as good as the data that was fed into the model. In fact, selecting the right data for your ML application is probably more important than selecting the right model.
This brings us to the necessity for a more data-centric AI, and by that I mean a machine learning process that focuses first and foremost on partitioning, selecting, and accurately labeling the data that we use to train these systems. For any AI system to add value both immediately and in an ever-changing environment, it must have high-quality data to train the models.
Too often we see naive applications of machine learning technology ignoring this fundamental requirement, leading to erroneous results, unintentional bias, and systems that are overly brittle when dealing with boundary cases.
High accuracy and a low level of expert intervention
By focusing on the quality of the incoming training data, the business can ensure that the machine learning algorithm continues to perform with high accuracy and a low level of expert intervention. This reduces wasted time for both the business and its customers.
The analogy from the physical world would be autonomous driving systems. Despite years of research, cars that safely drive themselves still seem to be a long way off. But driving assistance technology is already here and very effectively deployed on millions of vehicles. The key is to utilize the technology trained on a limited data set in a limited role.
AI research organizations would do well to spend less resources generating new content with AI and more resources on figuring out how to accurately and sustainably ingest existing content in a way that makes us all able to do our jobs better. Such comprehensive AI systems enable a man-machine partnership that furthers creativity rather than just mimicking it.
Time and again we as an industry repeat this mistake with regard to how to properly apply AI technology. We let our desire for the cool and revolutionary application blind us to the obvious practical use. Let’s all finally learn this lesson and get it right this time around—AI does best when it assists us, it fails miserably when it tries to replace us.
About the Author
Scott Spangler is a named IBM Distinguished Engineer, former IBM Watson Health Researcher, Chief Data Scientist, and the author of the book ‘Mining the Talk: Unlocking the Business Value in Unstructured Information.’ He holds a bachelor's from MIT in Math and a Master's from the University of Texas in Computer Science. He has over twenty years of experience designing and building solutions for unstructured data, and over ten years focusing specifically on life sciences and drug discovery.
Spangler’s areas of interest include unstructured text analytics for scientific discovery, data analysis, statistics, design of experiments, Java application development, intellectual property analysis, and corporate brand reputation analysis. He holds more than 50 patents and has written over 50 peer-reviewed scientific articles. He is currently Senior Data Scientist and Principal Consultant at DataScava.