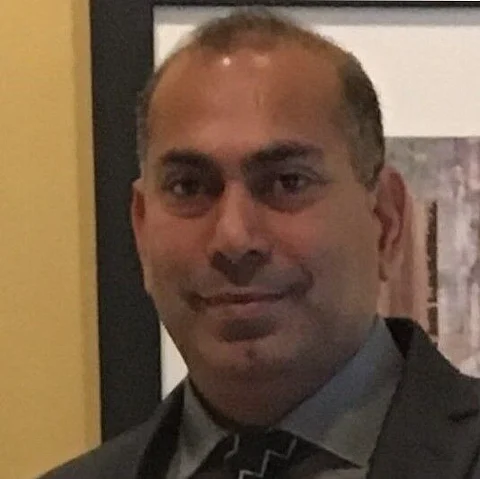
Karan Dhawal, Enterprise Data & Transformation Strategy Leader | ZS
What does it mean to be data-driven? A data-driven approach enables companies to examine, collect, organize and leverage their internal and/or external data, serving their customers better. A data-driven organization makes strategic decisions based on data analysis and interpretation at four analytics levels: descriptive, diagnostic, predictive, and prescriptive.
In this article, we review what it really means to be data-driven and look at a potential framework to measure data-driven culture.
We have formal processes to measure enterprise data management maturity, such as the Data Management Maturity index. EDM Council members have developed the Data Management Capability Assessment Model (DCAM) to score end-to-end enterprise data management journeys. The DCAM model categorizes the maturity journey into six levels: Not Initiated, Conceptual, Developmental, Defined, Achieved, and Enhanced.
It is usually hard to infer by using DCAM or a similar model that a company at Level 6 on a data maturity index is more data-driven than a company on Level 4 of a data maturity index.
A data-driven culture is NOT a Big Bang-type event. It is a journey. The framework is a way to measure progress toward becoming data-driven. Data strategy, data literacy, organizational change management, data talent, and leadership are all key maturity aspects needed for this journey.
A mature, data-driven company will have data architecture guidelines, including mature master data management, data replication, data privacy, and data security infrastructure. Business users can access the data they need, work with the data tool of choice, get insights from the data, and then communicate at all levels using data storytelling.
It becomes challenging to measure this intangible data-culture behavior. The proposed potential framework below allows us to assess “data-driven” at four levels that require top-down, executive-level commitments.
1. Key Business Questions
Does the company focus on key business questions (KBQs) and have these questions documented by the department? These KBQs need mapping and tracking for all investments within that department and should be linked to key business outcomes at the enterprise level.
2. Key Business Processes
Does the company have a regular cadence to identify, document, and update key business processes? Does everyone involved in the process understand the underlying data that answer the key business questions by departments?
Usually, a key business process is directly linked to the Level 1 business process.
Example: In Level 1, marketing and selling products and services business process identification and syndication of customer master will be considered as a critical Level 2 business process. Managing links and information from customer quarterly and annual reports will not be regarded as a key business process.
3. Available Key Data
This step in the framework calls for detailed analysis — at the granular level — of the available data needed to support the key business processes identified at the department and enterprise levels. This step supports the WHY and WHAT questions: Why do we have this data? What additional data do we need, externally or within the company, from other business processes?
Key data directly supports key business processes. From our example above, customer master, customer hierarchy, and customer historical orders are vital data. Data from customer quarterly and yearly reports on P&L will not be considered key data for the identified key business process of marketing and selling products and services.
This level of analysis also provides visibility into data input controls. This is the most critical step to improve maturity toward a data-driven culture and is based on one of the foundational Lean Six Sigma principles to remove variation.
This step focuses on controlling the inputs via the identified business processes to improve data quality exponentially. Focusing on data validations for critical data elements, monetized or monetizable data elements, and business KPIs presents the best opportunity for trust-based, data-driven decisions. Enable and monitor data validation dashboards at the key business process, department, and enterprise levels.
4. Executive Decision Traceability Matrix
The company has an executive decision traceability matrix that gathers information across enterprise and business lines. What decisions are being made? Who makes those decisions, and what data and processes are critical to those decisions? At what level are the decisions made, and which ones roll up to the next level? What is the accuracy of data quality needed for those decisions, etc.?
Information on historical decision making and key business questions, processes, and data allows for laser-focused investments in data initiatives and enterprise transformation efforts.
This framework leverages a data community that is inquisitive, iterative, and innovative in bringing value toward business goals. Data fluency will still need the appropriate level of data governance, data quality, privacy, and security, along with data risk dashboards for all decision-makers across the company.
In summary, four simple steps are part of a framework providing a quick way to review data-driven maturity levels across the organization. These four levels or milestones in the journey — Key Business Questions, Key Business Processes, Available Key Data Enterprise, and Decision Dashboard — work across data lifecycle management processes.
About the Author
Karan Dhawal is a passionate strategy and delivery leader in enterprise digital and data culture transformation. He specializes in designing technology and professional services solutions to solve complex business challenges related to data architecture, cloud computing, and big data platforms.
Under Dhawal’s tenure, companies such as Chevron, Covance, American Family Insurance, Sentry Insurance, Telephone & Data Systems, Owens-Illinois, and Rockwell Automation evolved from data-siloed to data-driven. His passion for creating innovative data products allowed these companies to generate more revenue through mergers and acquisitions, data-sharing-enabled growth strategies, and SaaS-led enterprise transformations.
Dhawal graduated from the Chief Data Officer Certificate Program at Carnegie Mellon University and the Artificial Intelligence: Implications for Business Strategyexecutive courseat the Massachusetts Institute of Technology.